The AI Revolution: Reshaping the Infrastructure Landscape
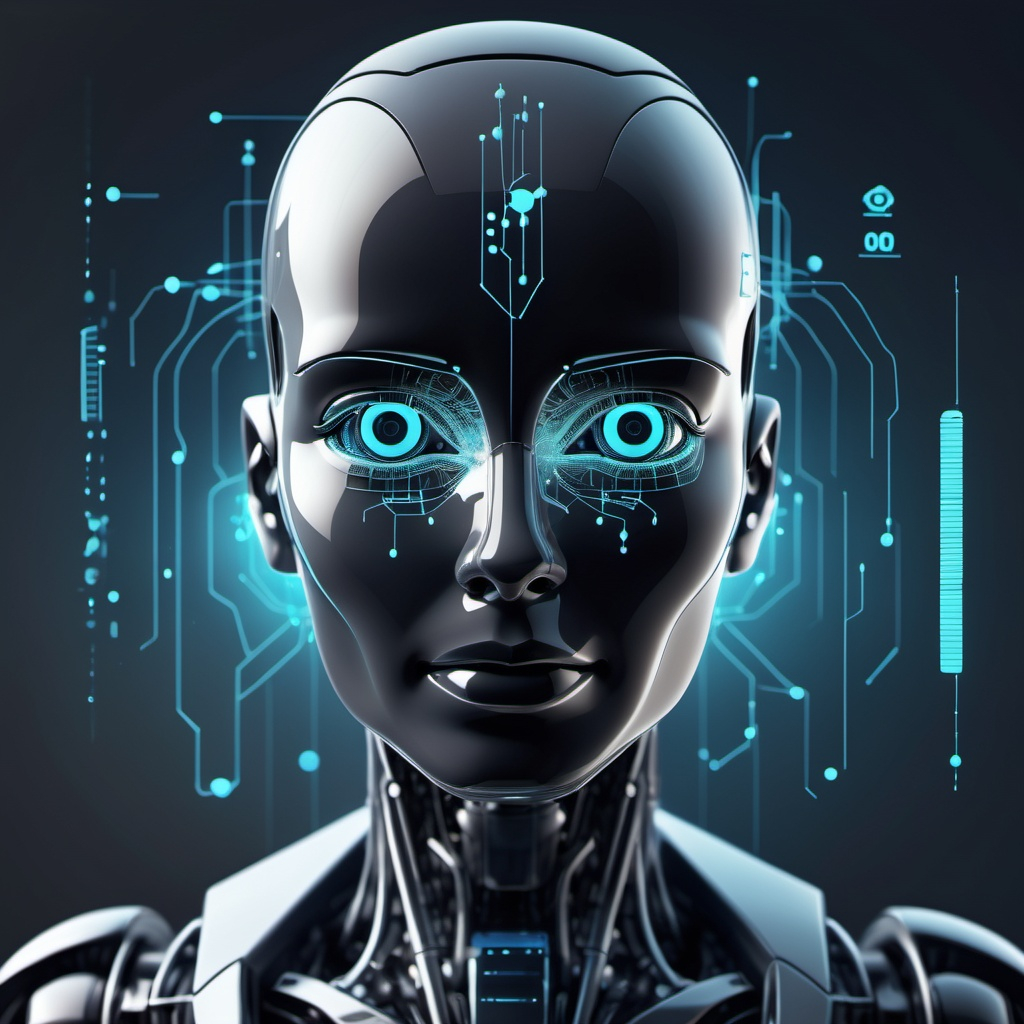
A new AI-focused infrastructure is emerging to drive the next wave of enterprise data software. Building on a history of infrastructure investments - from chip and semiconductor innovators like Habana and Intucell, to developer platforms like Twilio and Auth0, and modern data tools like HashiCorp and Imply - this shift marks the arrival of new infrastructure tools designed specifically for enterprises in the AI era.
Data Growth: Meeting AI's Expanding Needs
Since the 2017 breakthrough paper "Attention is all you need," which established the foundation for transformer deep learning architecture, we have entered a Cambrian explosion of AI research. New papers are being published daily, compounding at an astonishing pace.
The AI revolution is fundamentally reshaping the data infrastructure landscape across many critical vectors.
First, AI is becoming the beating heart of modern data stacks. Incumbent infrastructure providers aren't just watching from the sidelines - they're actively weaving AI capabilities into their core offerings, enhancing everything from data synthesis to retrieval and enrichment. Recognizing AI's strategic value, many have gone a step further, launching dedicated products for AI workloads and AI-first customers. Take database companies, for instance: they're now treating embeddings as first-class citizens, either integrating them as features or spinning up standalone solutions.
Second, we're witnessing an unprecedented fusion of data and AI. The sheer scale of data growth is staggering - projections show generated data soaring to 612 zettabytes by 2030 (that's one trillion gigabytes per zettabyte), driven largely by ML/AI advances and the explosion of synthetic data across all modalities. This isn't just about volume - it's about mounting complexity and diversity in data types and sources. In response, companies are pushing the boundaries of hardware innovation: developing more muscular processors like GPUs and TPUs, engineering sophisticated networking hardware for seamless data movement, and pioneering next-generation storage solutions.
Finally, while a new breed of AI-native and AI-embedded startups is emerging - companies either built on AI/ML foundations or using it to supercharge existing capabilities - they're facing a significant challenge. Current data infrastructure remains stubbornly misaligned with AI requirements. It's like trying to fit a square peg into a round hole, forcing AI engineers to cobble together workarounds and makeshift solutions within existing systems. This gap between traditional infrastructure and AI needs represents both a challenge and an opportunity for innovation.
Building the Foundation: Key Areas of AI Infrastructure Innovation
1. Model Architecture and Scaling: The race is on to develop more efficient, powerful, and specialized AI models. From improvements in transformer architectures to entirely new paradigms like state-space models, researchers are constantly pushing the boundaries of what's possible.
2. Deployment and Inference Optimization: As AI moves from research labs to production environments, there's a growing focus on optimizing model deployment and inference. Innovations in hardware utilization, memory management, and serverless infrastructure are making AI more accessible and cost-effective.
3. Advanced Training Techniques: The AI community is exploring a wide range of techniques to enhance model performance, from novel fine-tuning methods and retrieval-augmented generation to innovative prompting strategies and model mixing approaches.
4. DataOps 2.0 in the age of AI: Traditional data management practices are being reimagined to cater to AI workflows. This includes new approaches to data curation, storage (like vector databases), and annotation, as well as tools for handling unstructured data at scale.
5. AI Observability and Monitoring: As AI systems become more complex and mission-critical, there's a growing need for sophisticated monitoring and observability tools. These solutions aim to provide insights into model performance, detect anomalies, and ensure regulatory compliance.
6. AI Development Orchestration: To manage the increasing complexity of AI development workflows, new orchestration tools are emerging. These platforms aim to streamline the AI development lifecycle, from experiment tracking to model deployment and beyond.
The AI Infrastructure Boom: A New Frontier for Innovation and Growth
As we stand on the center of an AI revolution, a significant opportunity is emerging for those who can provide the foundational tools and infrastructure necessary to make AI operational at scale. Just as the gold rush created fortunes for those supplying miners with essential equipment, we anticipate that the companies building the backbone of AI infrastructure will become the tech giants of tomorrow.
At NeurochainAI, we specialize in providing turnkey GenAI infrastructure optimization solutions for enterprises, helping to reduce costs by up to 68%. Whether businesses want to manage their own infrastructure or prefer a fully managed service, we provide the tools, expertise, and infrastructure to support a wide range of AI needs.
Key services we offer:
1. Inference Routing Solution (IRS): Our proprietary IRS allows multiple AI models to run on a single GPU, reducing the total number of GPUs needed in an infrastructure. It also optimizes GPU fill rates to meet desired capacity, whether it's 100% or less. Additionally, AI model optimization through quantization reduces the precision of numbers in neural networks without sacrificing accuracy, resulting in smaller, faster models that use less computational power.
2. Optimal Infra Set-Up And Management Solution: Managed Inference Infrastructure (MII): We offer a comprehensive solution for AI infrastructure, starting with a tailored setup based on your specific needs. Leveraging our network of GPU providers and cloud compute partners, we establish a custom infrastructure optimized by our proprietary Inference Routing Solution (IRS). This process involves careful selection and configuration to ensure peak performance. Once operational, our team takes on full management responsibilities, providing hands-on oversight of your AI systems. This end-to-end service allows businesses to harness the power of AI without the need for in-house expertise, ensuring optimal performance and efficiency while giving you peace of mind.
3. Cheap Public Network Solution: Distributed Inference Network: For enterprises that are not looking for a dedicated GPU infrastructure and require AI solutions where data privacy is not of concern, like customer support agents that use public data and similar, can benefit from the infinitely scalable Inference DePIN.
Future Outlook and Conclusion
The AI infrastructure paradigm represents one of the most significant shifts in enterprise technology in recent years. While uncertainties remain, the opportunities for innovation are vast. Companies that can navigate this complex landscape while delivering scalable, efficient solutions will likely emerge as the next generation of infrastructure giants. As we continue to witness this transformation, the ability to adapt to evolving requirements while maintaining focus on core value propositions will be crucial for success in this new era of enterprise software.